FlickrLogos
FlickrLogos
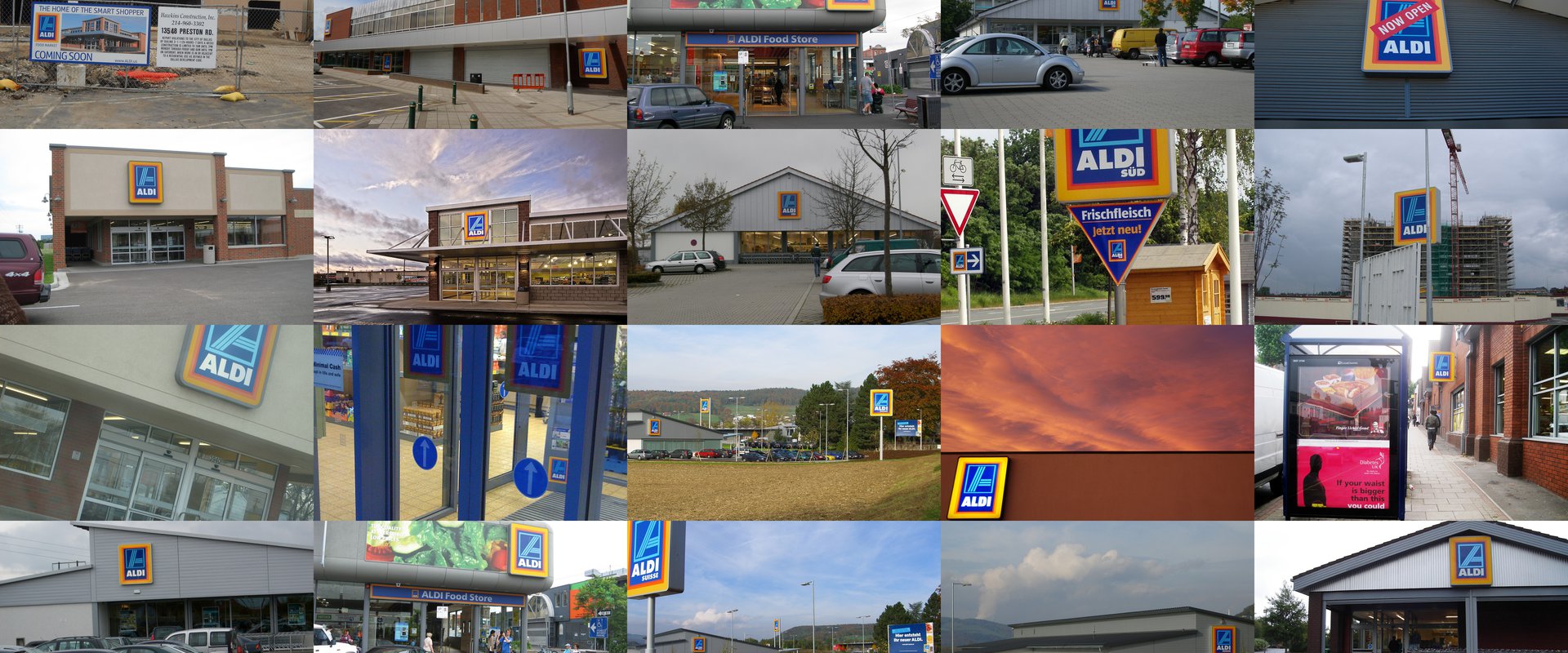
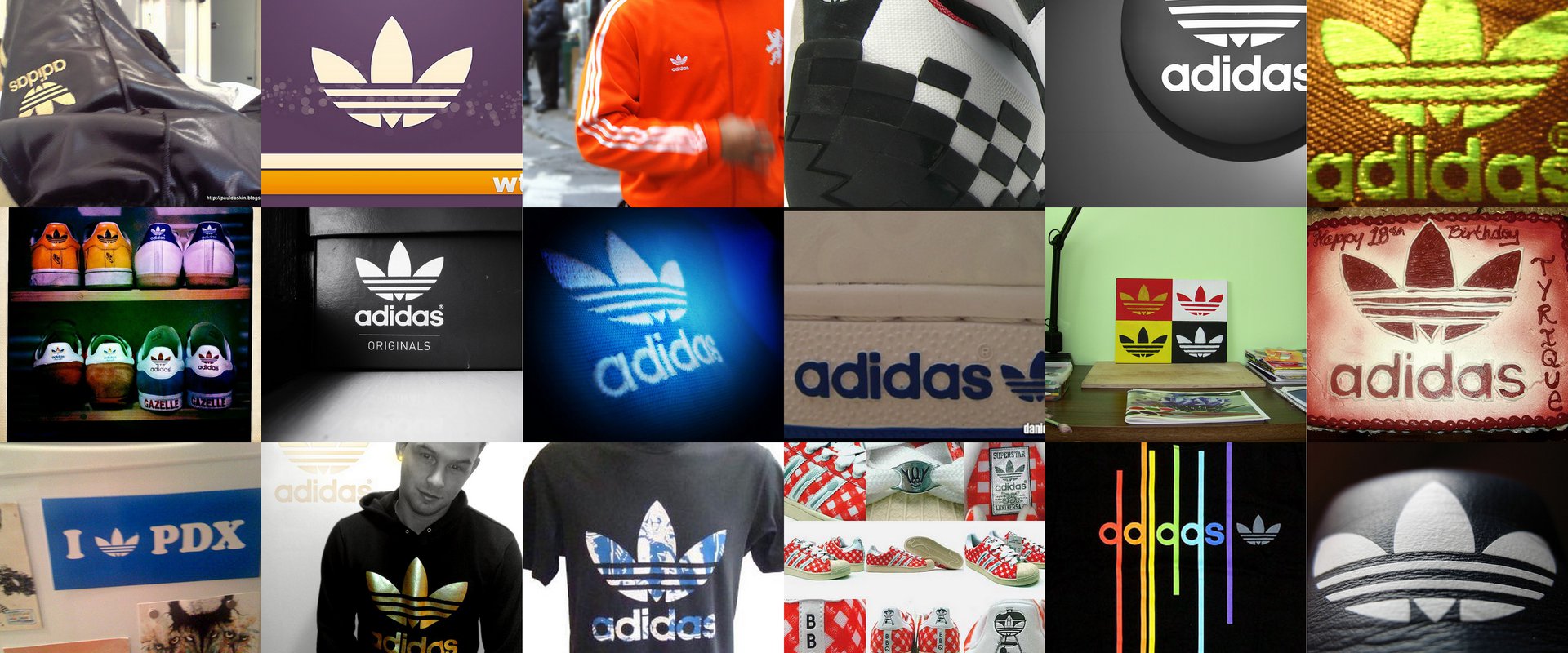
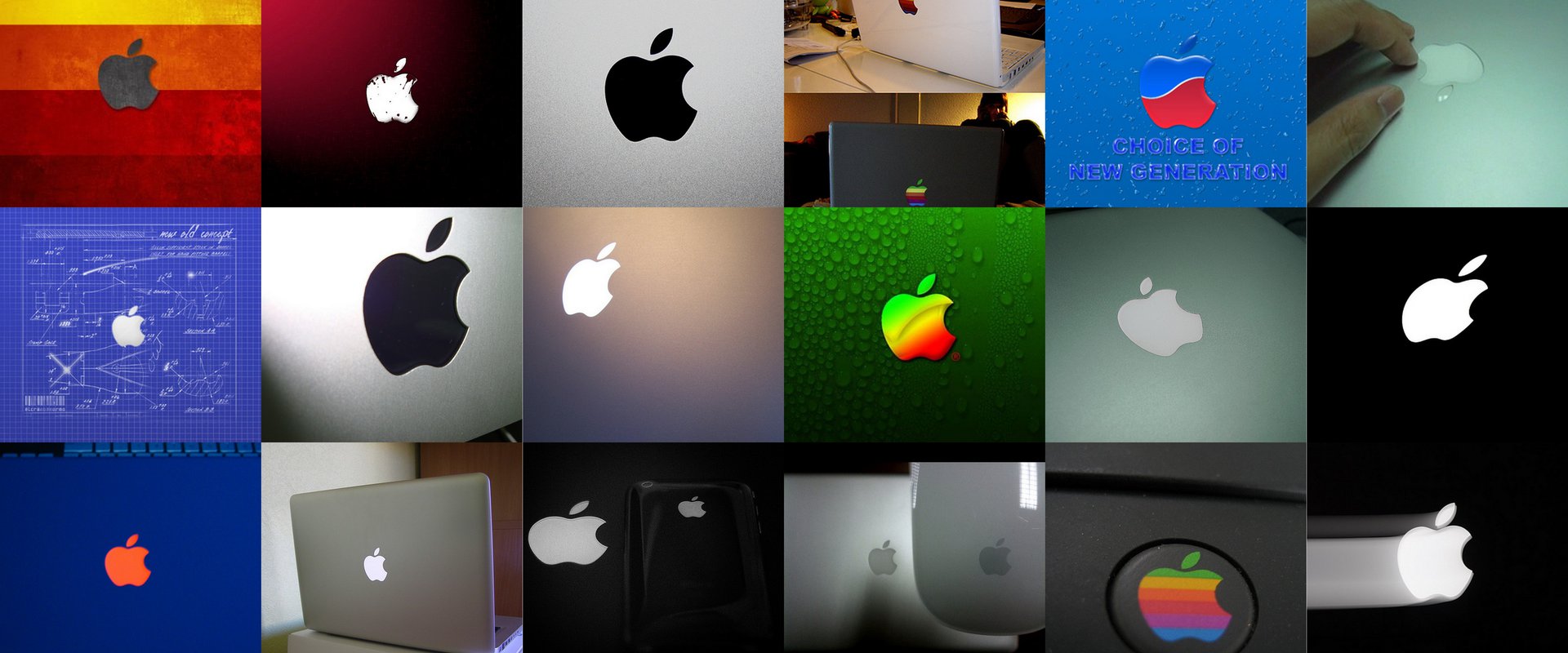
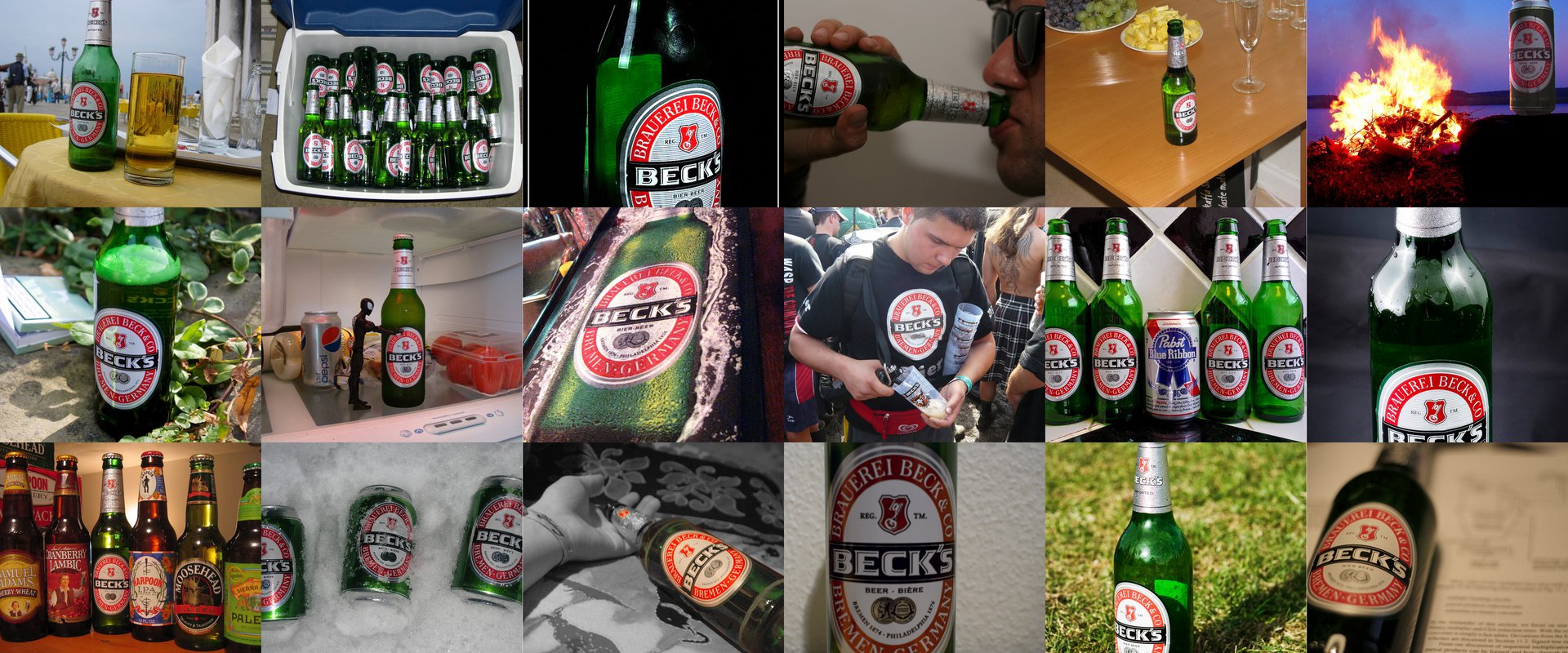
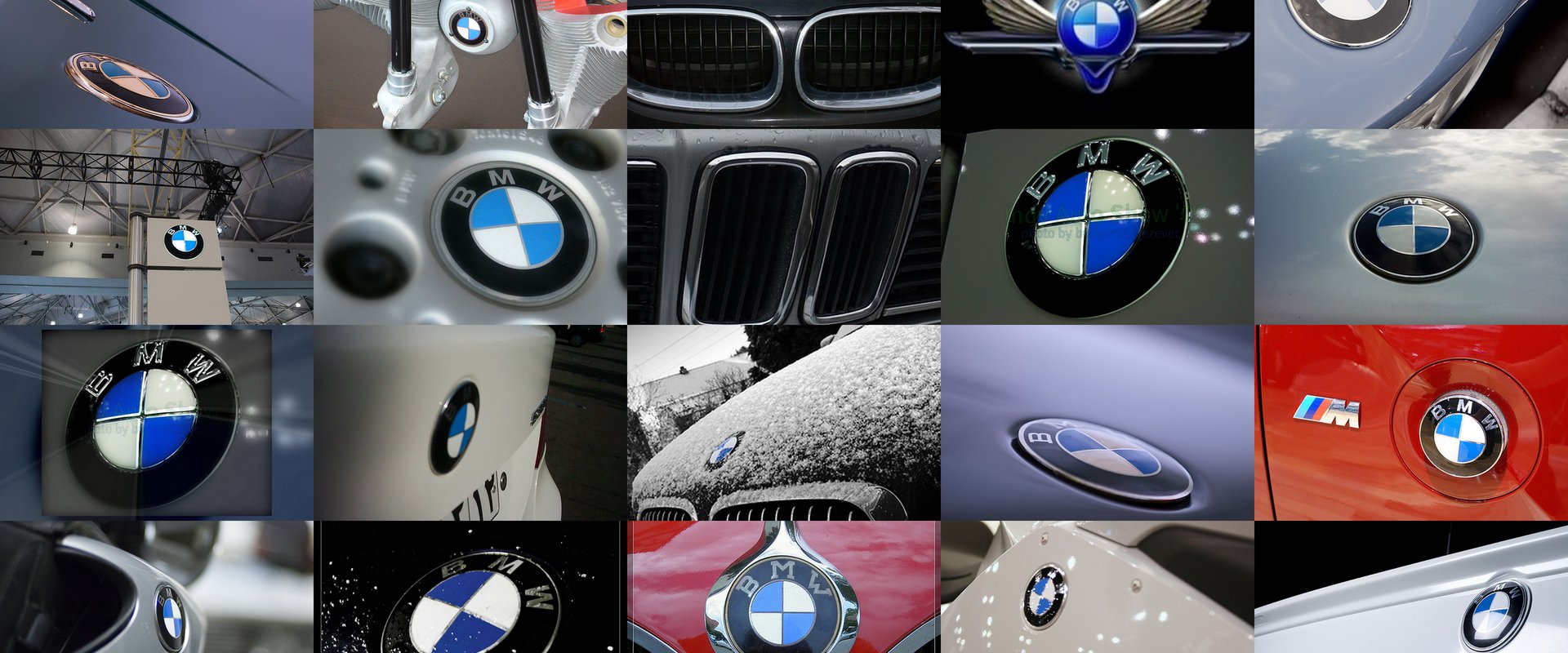
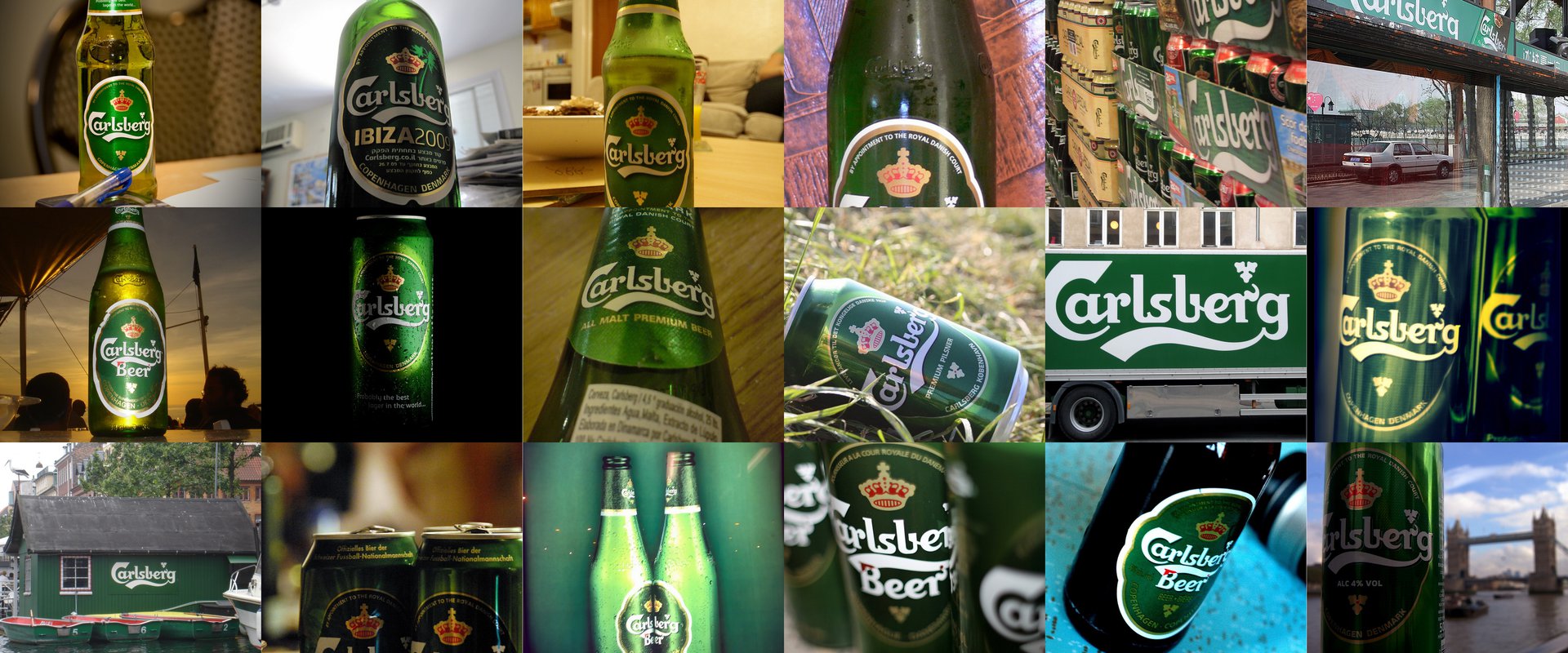
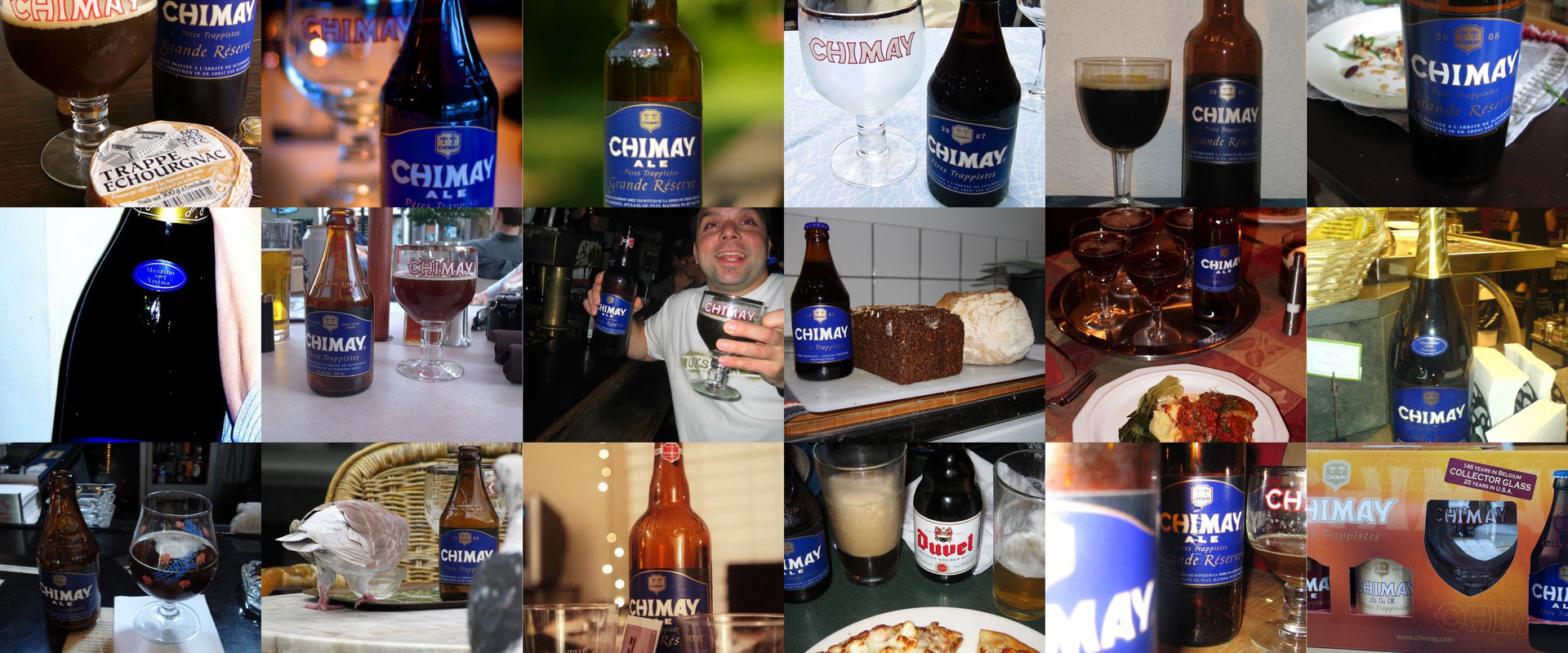
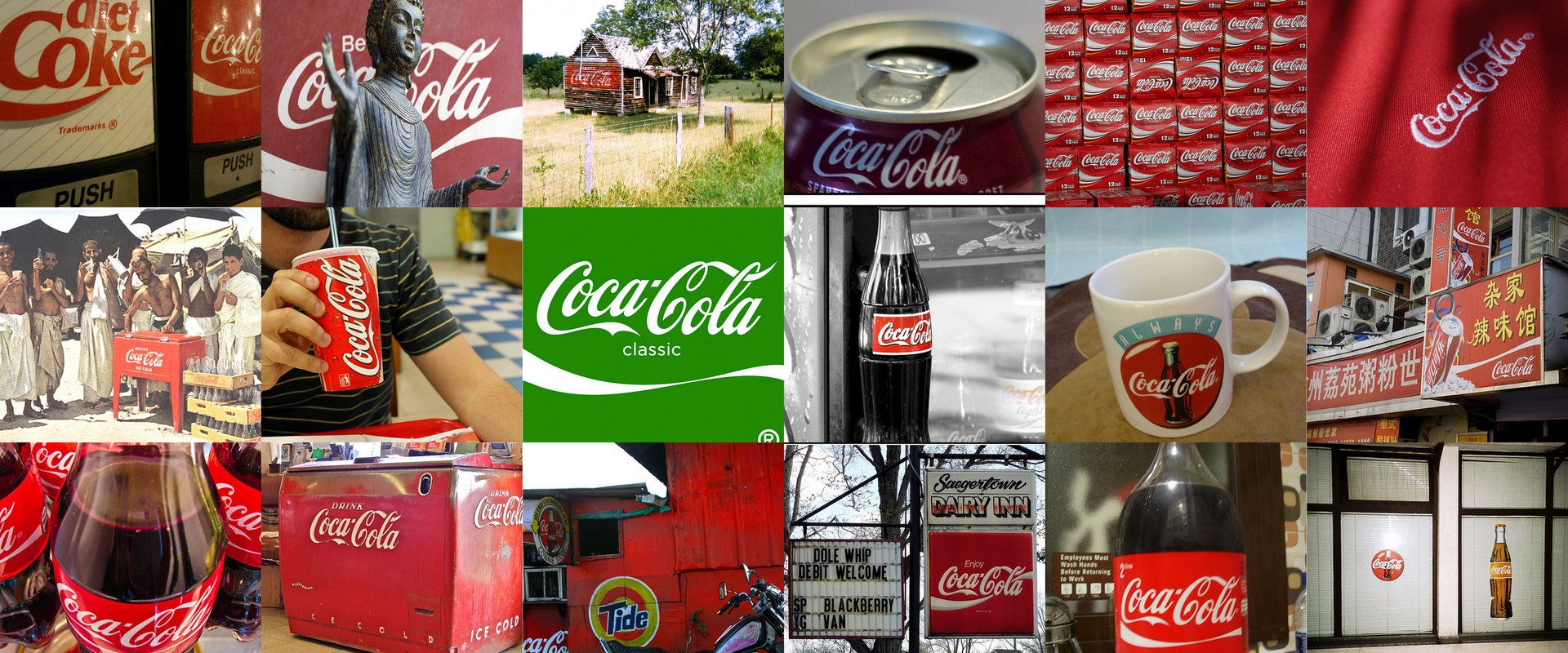
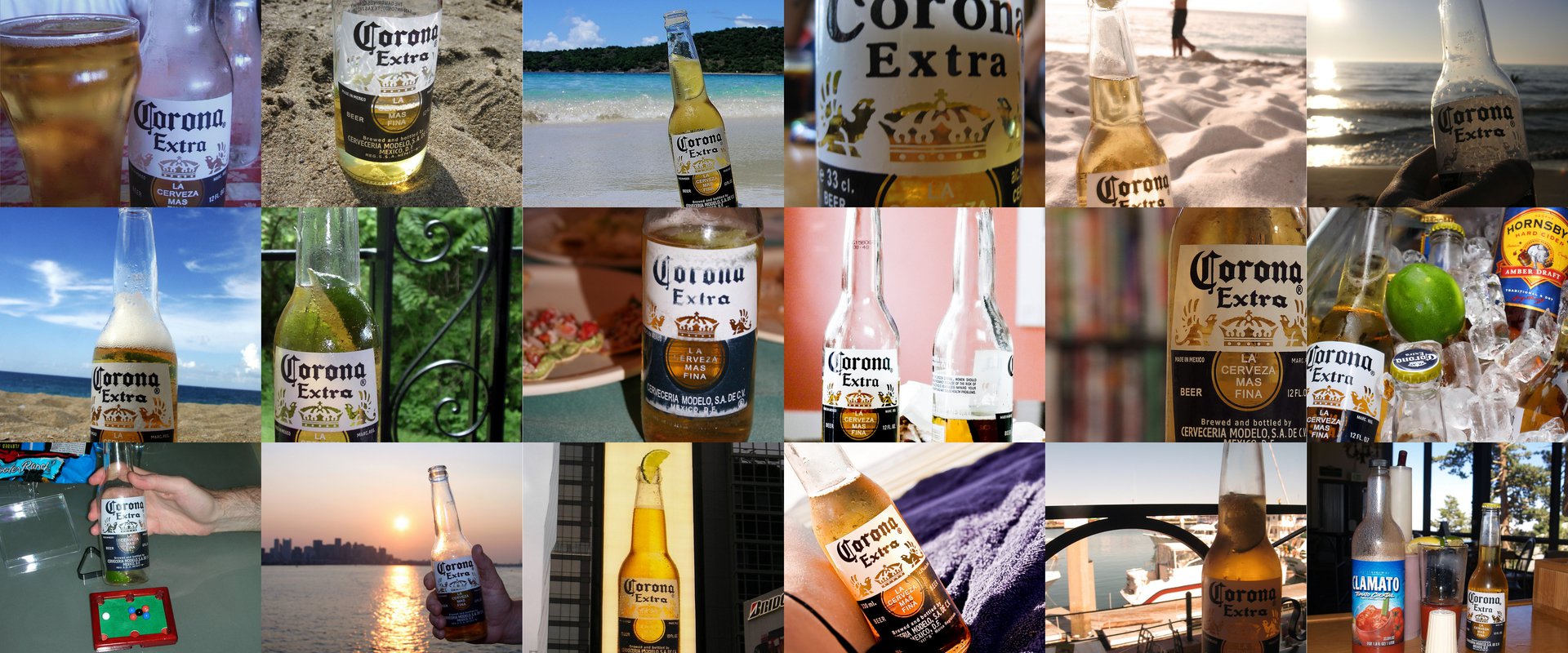
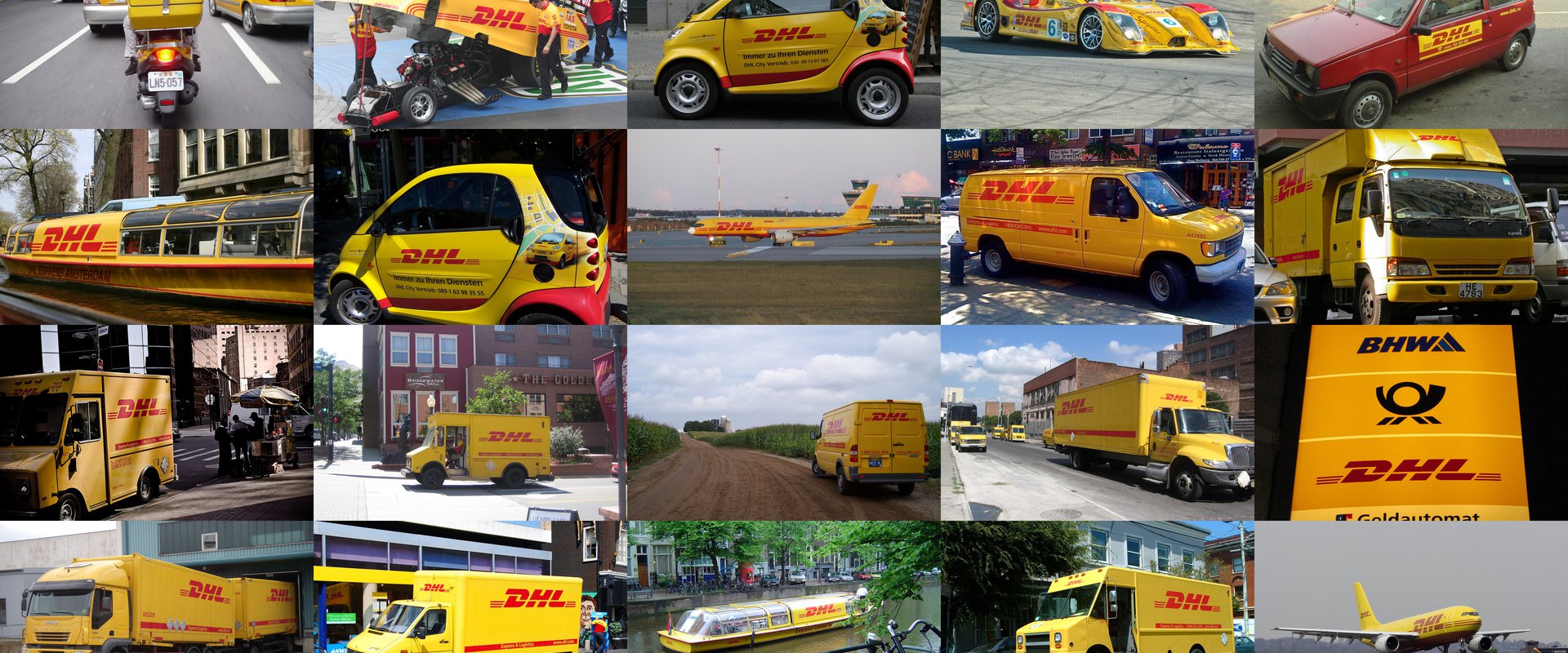
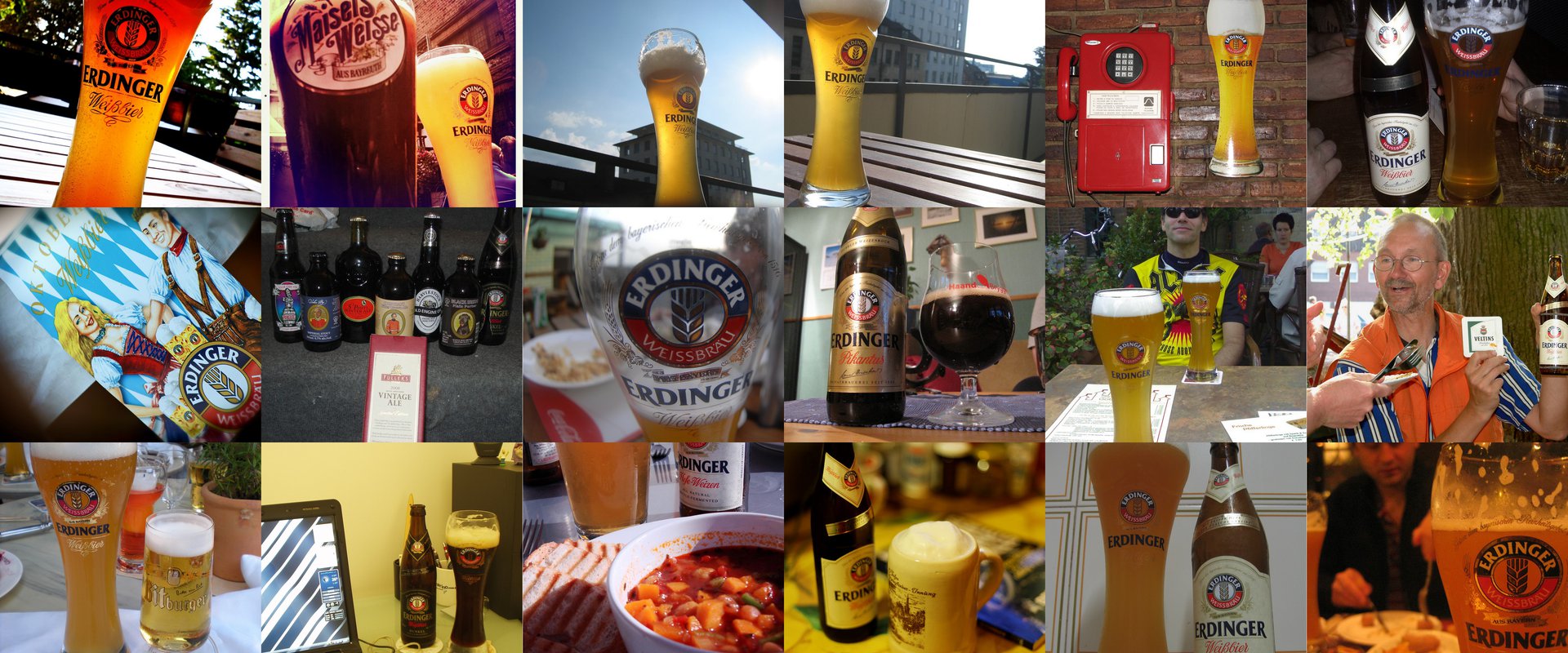
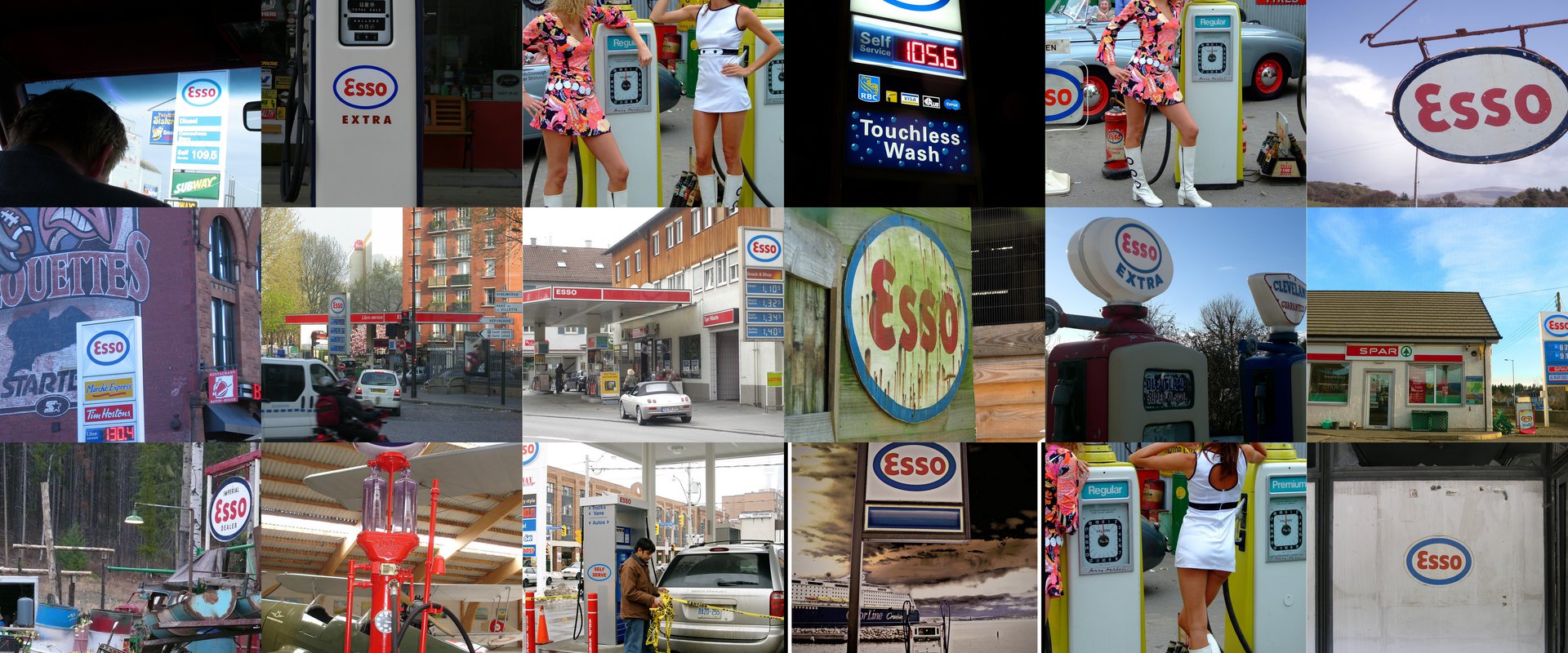
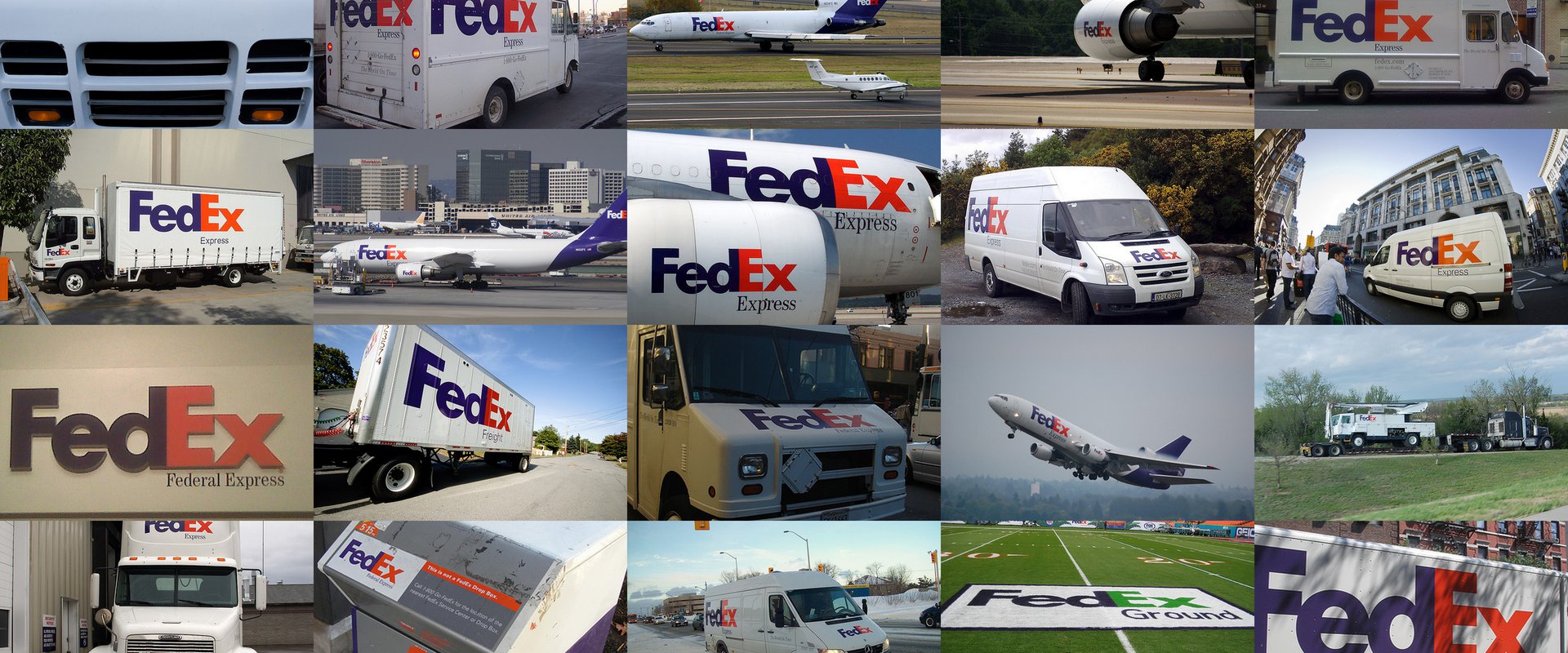
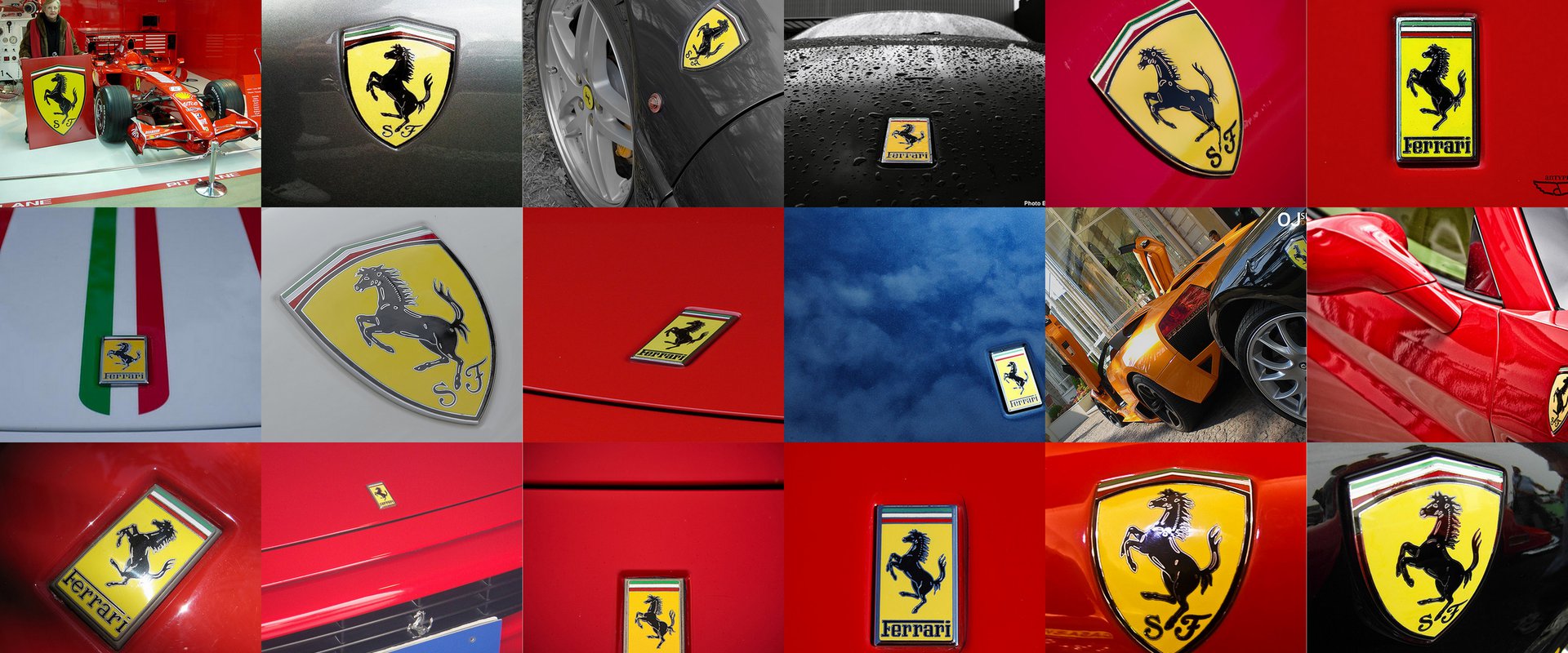
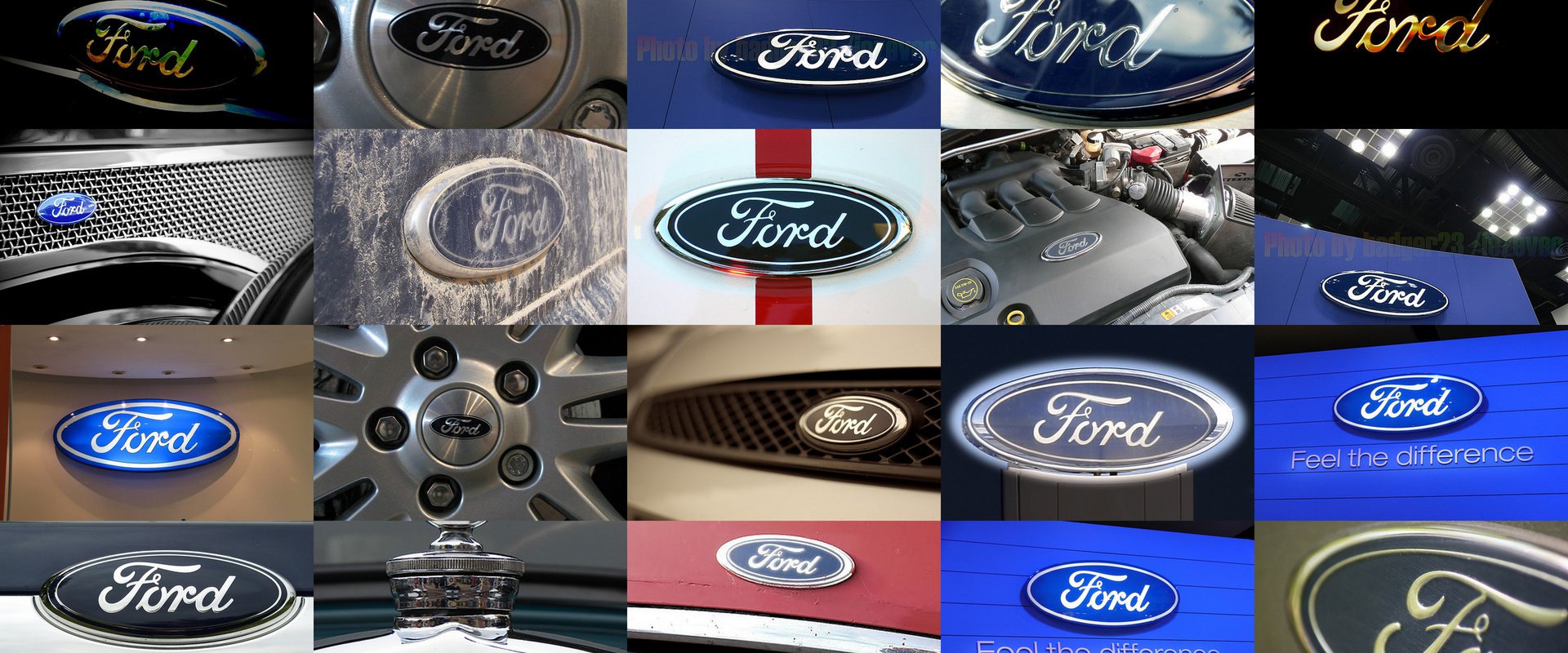
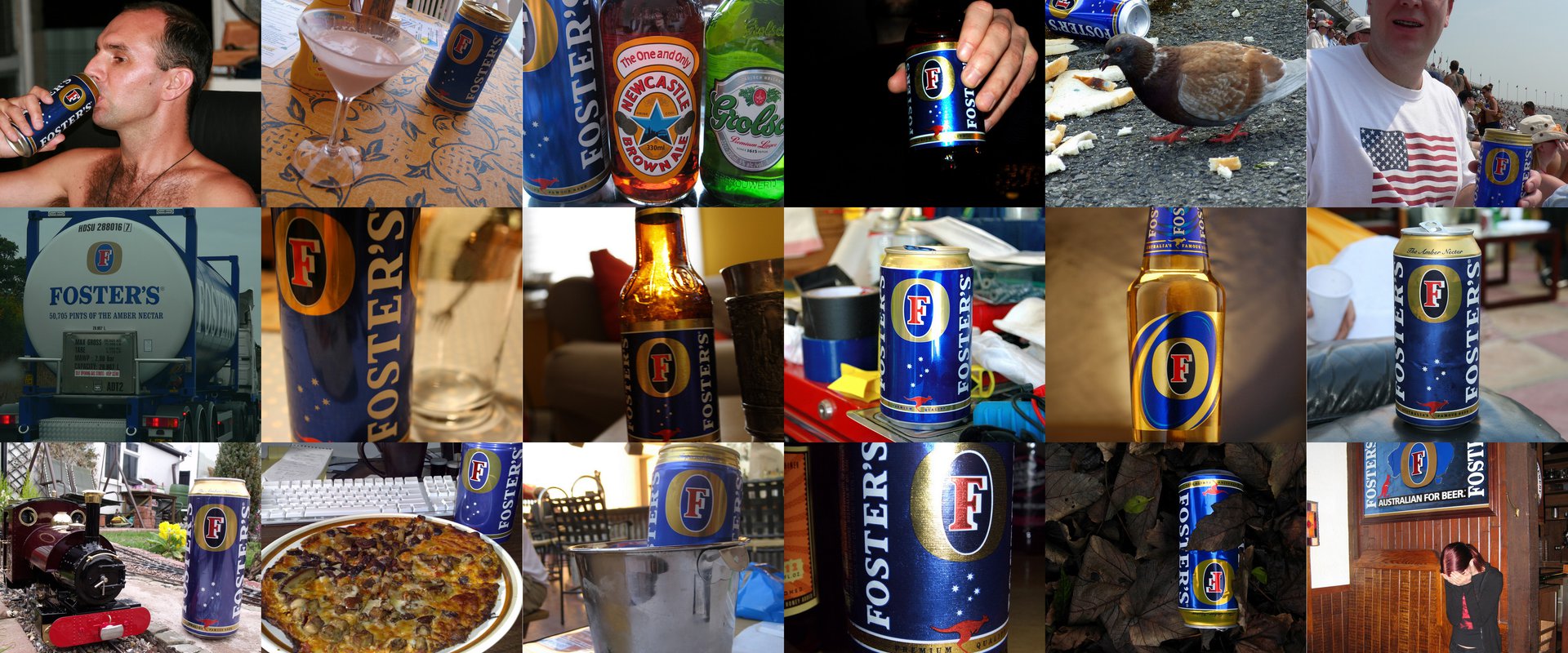
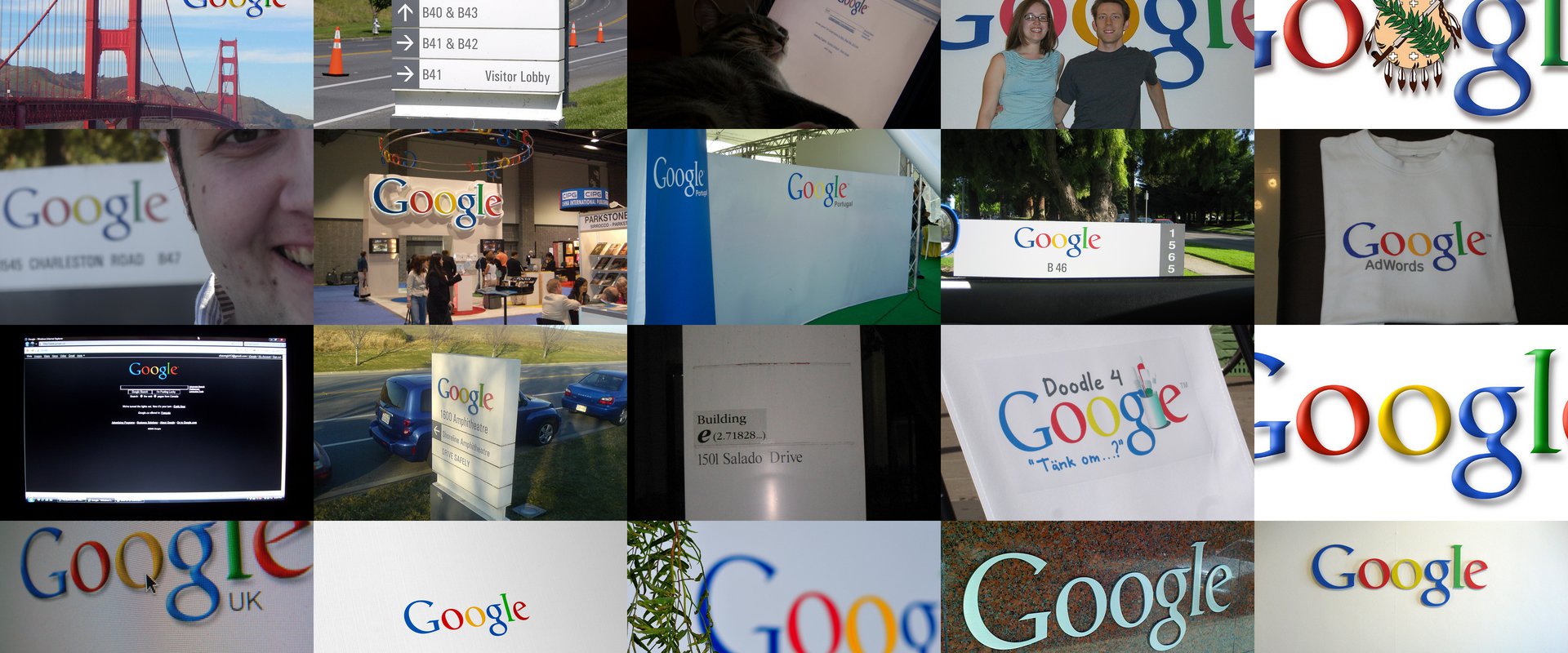
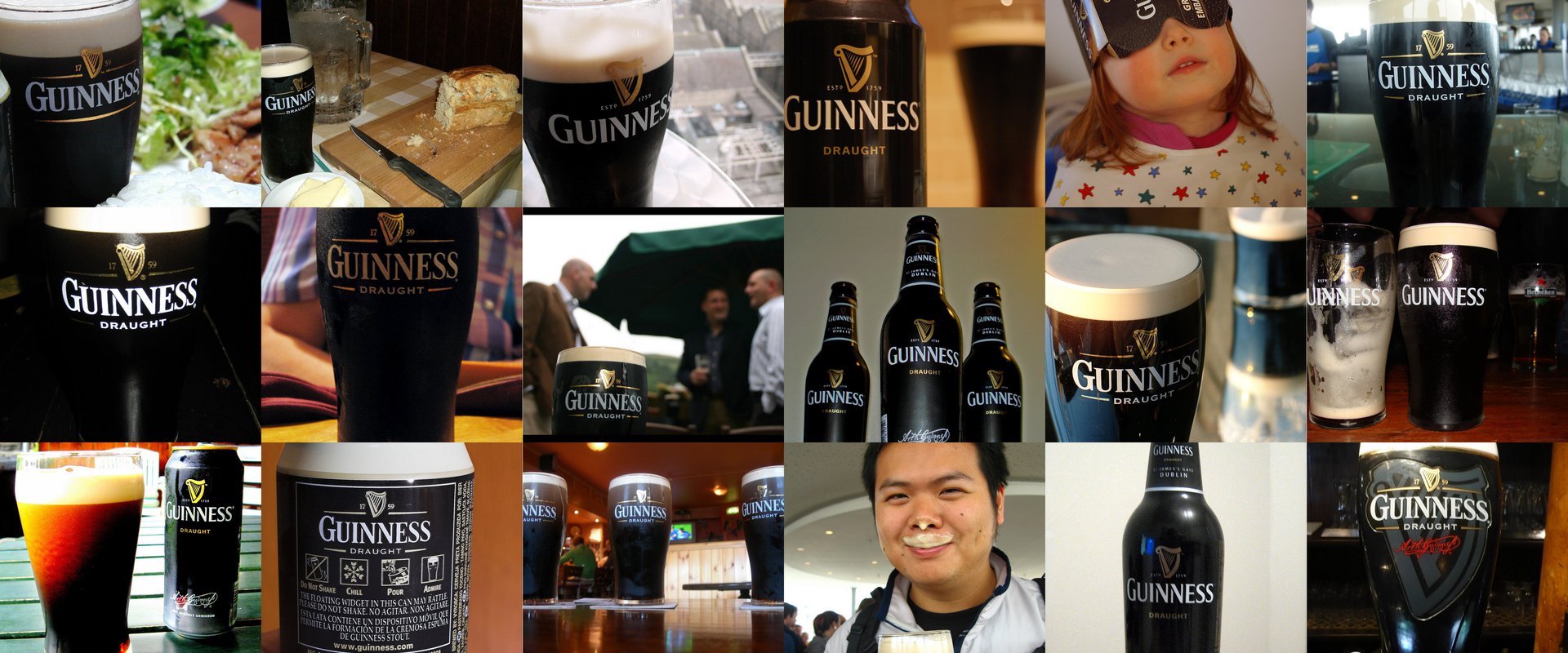
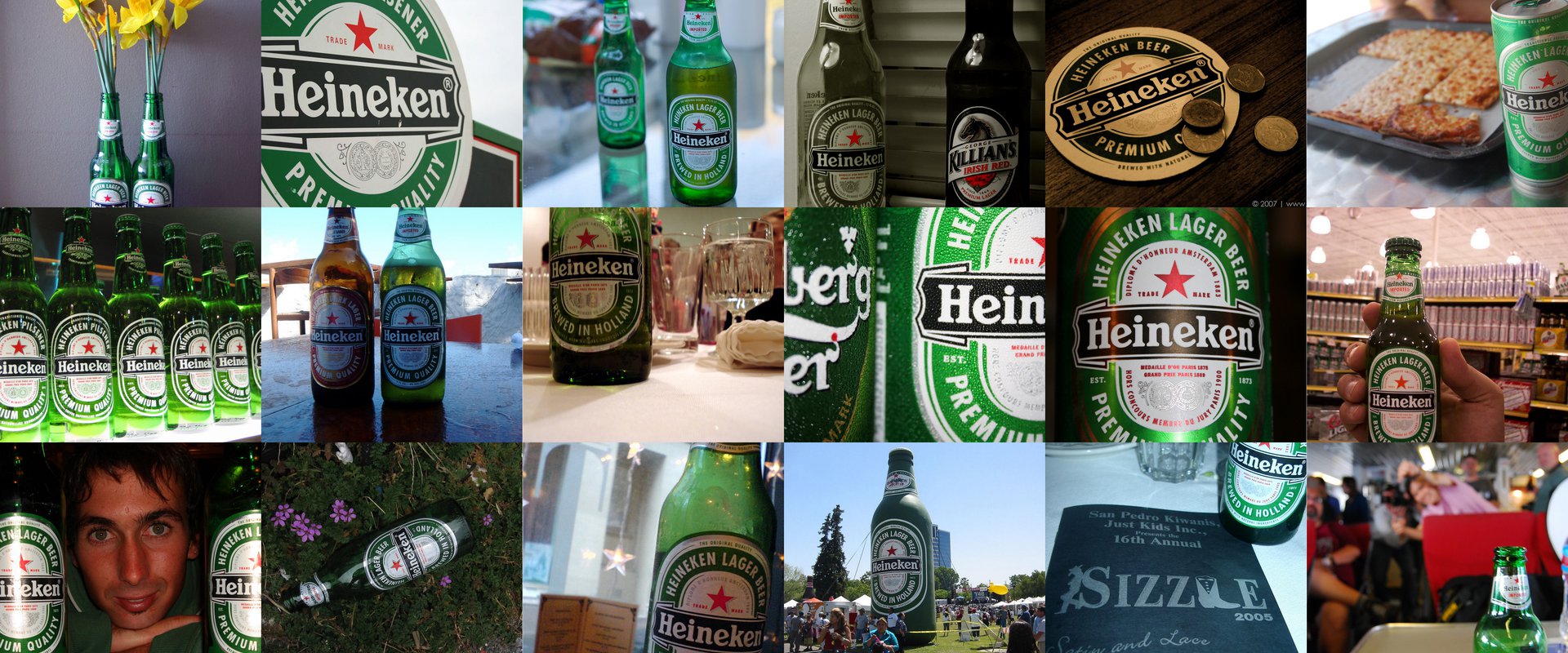
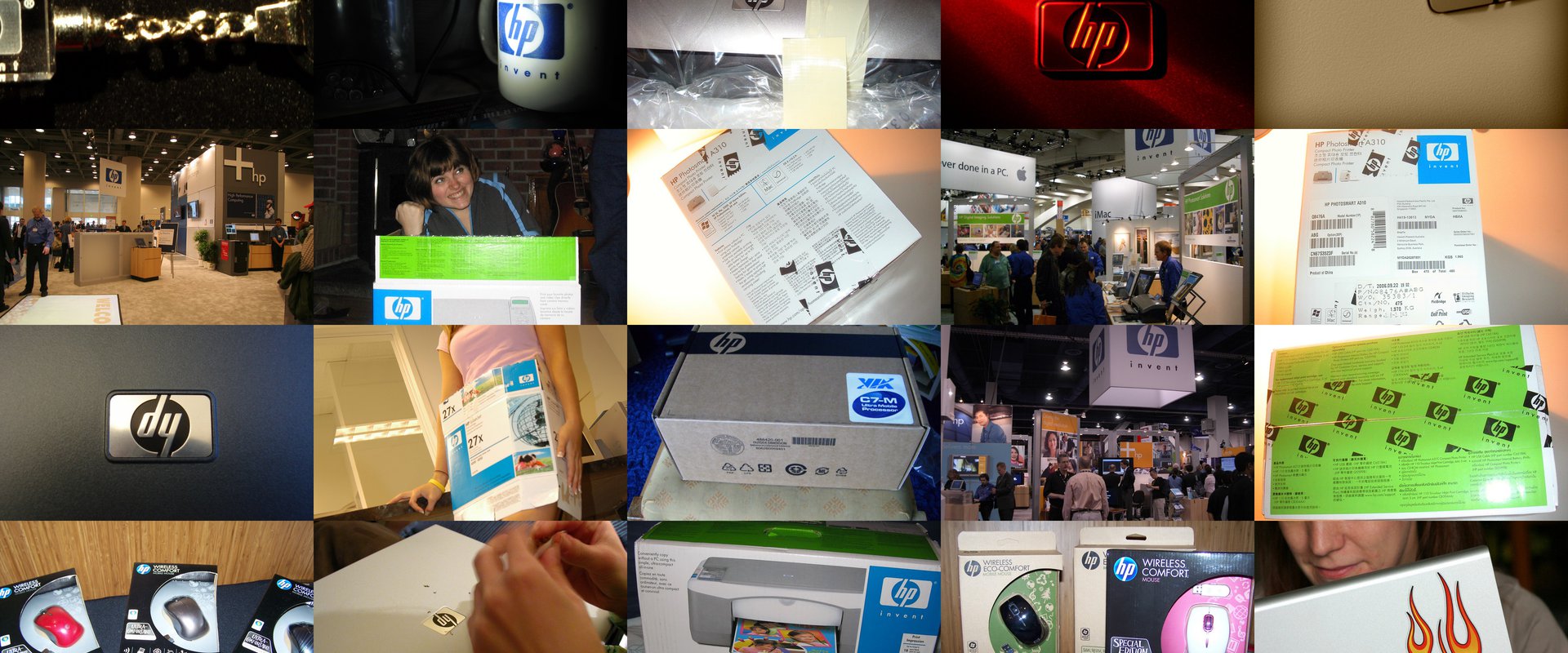
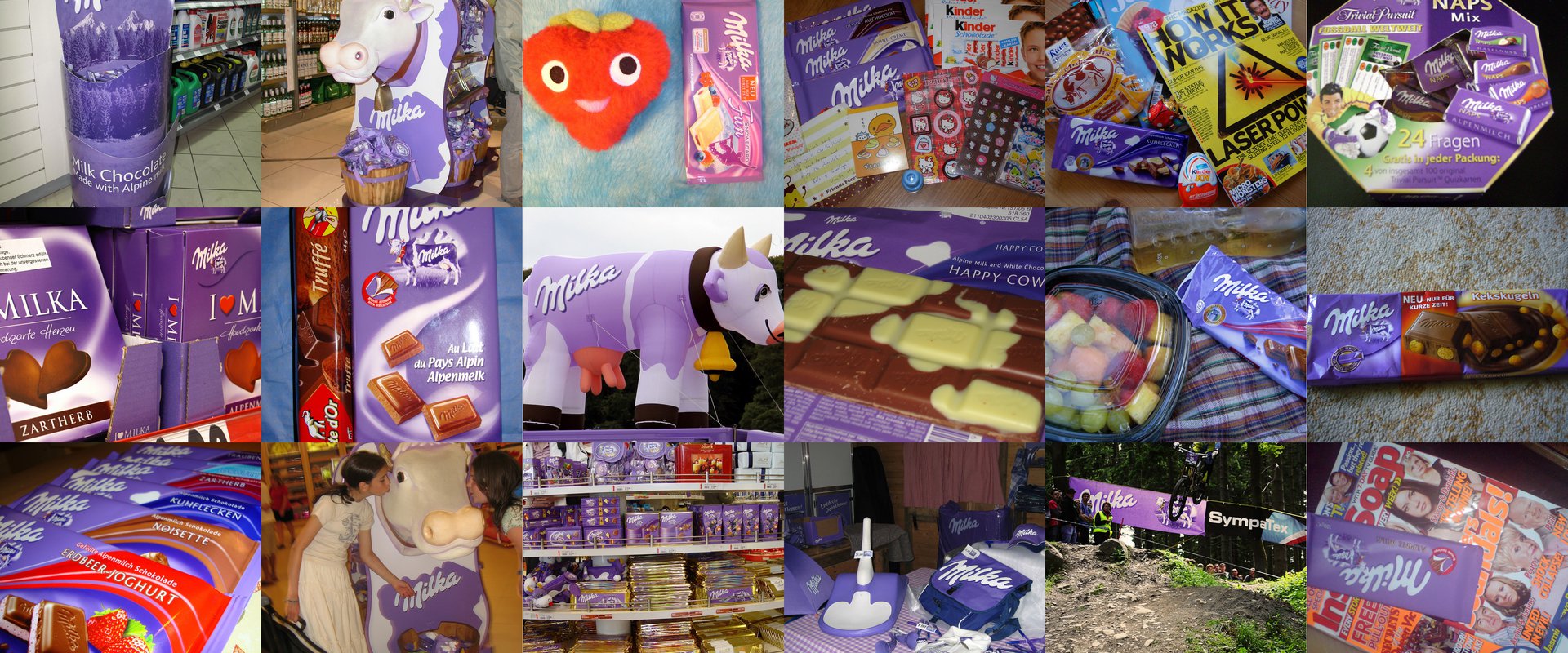
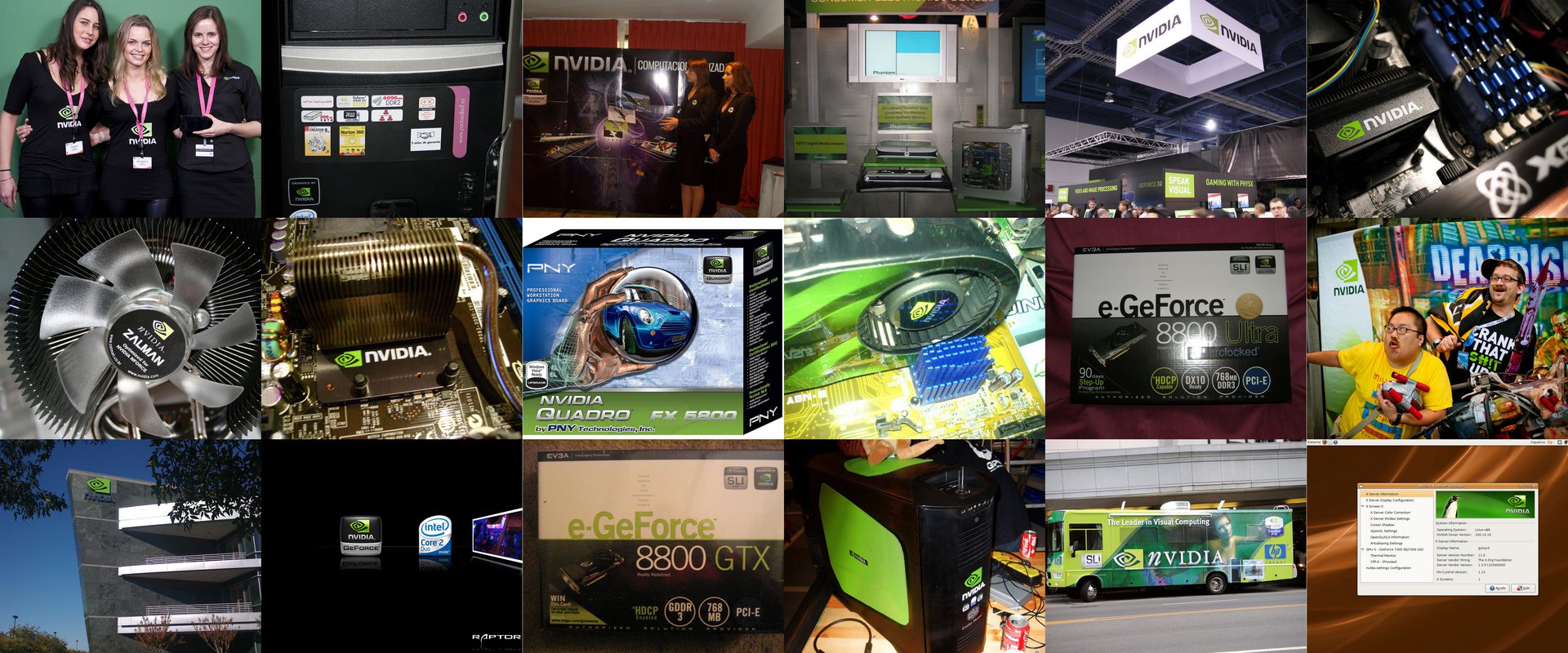
The FlickrLogos dataset consists of real-world images collected from Flickr depicting company logos in various circumstances. The dataset comes in two versions: The original FlickrLogos-32 dataset and the FlickrLogos-47 dataset. FlickrLogos-32 was designed for logo retrieval and multi-class logo detection and object recognition. However, the annotations for object detection were often incomplete,since only the most prominent logo instances were labelled. FlickrLogos-47 uses the same image corpus as FlickrLogos-32 but has been re-annotated specifically for the task of object detection and recognition. New classes were introduced (i.e. the company logo and text are now treated as separate classes where applicable) and missing object instances have been annotated. Furthermore, we provide corresponsing evaluation scripts.
FlickrLogos-32
Description:
The dataset FlickrLogos-32 contains photos showing brand logos and is meant for the evaluation of logo retrieval and multi-class logo detection/recognition systems on real-world images. We collected logos of 32 different logo brands by downloading them from Flickr. All logos have an approximately planar surface.
?
Classes:
There are 32 logo classes: Adidas, Aldi, Apple, Becks, BMW, Carlsberg, Chimay, Coca-Cola, Corona, DHL, Erdinger, Esso, Fedex, Ferrari, Ford, Foster's, Google, Guiness, Heineken, HP, Milka, Nvidia, Paulaner, Pepsi, Ritter Sport, Shell, Singha, Starbucks, Stella Artois, Texaco, Tsingtao and UPS.
?
Partitions / subsets:
The retrieved images were inspected manually to ensure that the specific logo is actually shown. The whole dataset is split into three disjoint subsets P1, P2, and P3, each containing images of all 32 classes. The first partition P1 - the training set - consists of 10 images that were hand-picked such that these consistently show a single logo under various views with as little background clutter as possible. The other two partitions P2 (validation set) and P3 (test set = query set) contain 30 images per class. Unlike P1 these images contain at least one instance of a logo but in several cases multiple instances.
?
To facilitate the development of high-precision classifiers the evaluation of their sensitivity on non-logo images is important. Therefore both partitions P2, and P3 include another 3000 images downloaded from Flickr with the queries "building", "nature", "people" and "friends". These images are the negative images and complete our dataset. A brief summary of the data subsets is shown in the table below.
?
Partition | Description | Images | #Images |
---|---|---|---|
P1 (training set) |
Hand-picked images |
10 per class | 320 images |
P2 (validation set) | Images showing at least a single logo under various views | 30 per class | 3960 images |
Non-logo images | 3000 | ||
P3 (test set = query set) | Images showing at least a single logo under various views | 30 per class | 3960 images |
Non-logo images | 3000 | ||
P1, P2 and P3 are disjoint. | ? | 8240 images |
?
![]() |
![]() |
![]() |
![]() |
![]() |
![]() |
?
FlickrLogos-47
Description:
The dataset FlickrLogos-47 contains photos showing brand logos and is meant for the evaluation of logo detection and recognition systems on real-world images. It is built from the same images than the FlickrLogos-32 dataset which have been re-annotated to fix mission annotations and to include more classes.
?
Classes:
There are 47 logo classes: Adidas (Symbol), Adidas (Text), Aldi, Apple, Becks (Symbol), Becks (Text), BMW, Carlsberg (Symbol), Carlsberg (Text), Chimay (Symbol), Chimay (Text), Coca-Cola, Corona (Symbol), Corona (Text), DHL, Erdinger (Symbol), Erdinger (Text), Esso (Symbol), Esso (Text), Fedex, Ferrari, Ford, Foster's (Symbol), Foster's (Text), Google, Guiness (Symbol), Guiness (Text), Heineken, HP, Milka (Symbol), Milka (Text), Nvidia (Symbol), Nvidia (Text), Paulaner (Symbol), Paulaner (Text), Pepsi (Symbol), Pepsi (Text), Ritter Sport, Shell, Singha (Symbol), Singha (Text), Starbucks, Stella Artois (Symbol), Stella Artois (Text), Texaco, Tsingtao (Symbol) Tsingtao (Text) and UPS.
?
Download
Download
If you wish to download one (or both of the datasets), please send an (informal) email to
request_flickrlogos@informatik.uni-augsburg.de. Please state your name, your institution and why you would like to have access to this dataset (we are curious). We then send you a download link by e-mail.
Note: This dataset consists of images downloaded from Flickr. Use of these images must respect Flickr's terms of use.
?
Important Notes
There is a similar dataset called FlickrLogos-27. It consists of 27 classes only and while there is some overlap it is largely different from our FlickrLogos-32 dataset. Results for these different datasets are not comparable.
?
Contact
If you have any questions, corrections or other issues please contact Stephan Brehm. The former maintainers were Christian Eggert and Stefan Romberg.